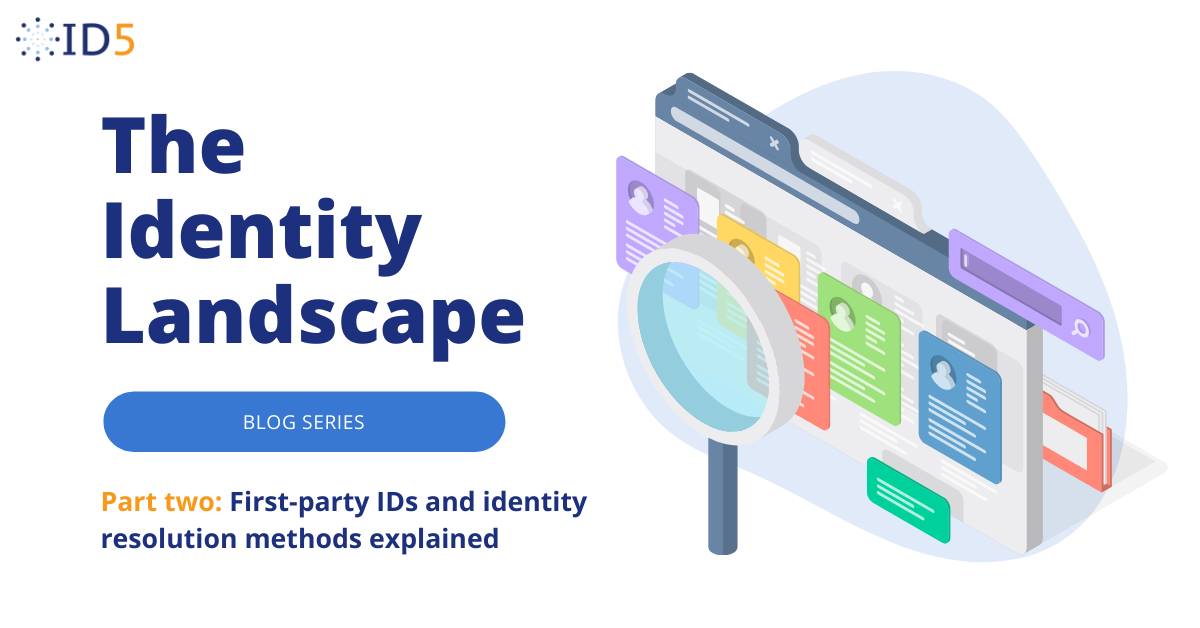
First-party IDs and identity resolution methods explained
- Posted by Anissa Connor
- On Mar 24, 2022
The digital advertising ecosystem is heavily reliant on conventional identification tools that are set to be blocked soon. Ad tech platforms have built their technology on third-party cookies and mobile ad IDs to enable brands to deliver personalized experiences to their audience. Third-party cookies and MAIDs have been used as deterministic signals to identify, track, target and measure user interaction across domains and devices.
The proliferation of devices and new digital environments has made it increasingly complex for brands to understand and interact with their customers. Today, people engage across multiple touchpoints throughout the customer journey. However, most of them are not logged in on every device. Therefore, the demise of the third-party cookie and MAID is making it more and more difficult for brands to understand how their customers interact and, subsequently, deliver relevant experiences.
First-party IDs
The upcoming sunset of third-party cookies and MAIDs has pushed the industry to find reliable alternatives to those identification tools. First-party user IDs have been created to provide identification capabilities and enable campaign strategies such as targeting, frequency capping and measurement without leveraging third-party cookies or mobile ad IDs.
However, it’s important to notice that not all first-party identifiers are created in the same way and to serve the same use cases.
Publisher-based identifiers: Publisher-based first-party IDs, such as SharedID by Prebid, are specific to the digital property they are set on. They do not offer any cross-domain or cross-device user recognition so their value is limited to helping the buy-side target, optimize and measure campaigns on a single domain or publisher.
Universal identifiers: First-party universal IDs attempt to resolve user identity across domains and devices but have to rely on other signals to achieve this successfully. Two identity resolution methods have emerged to help brands reconcile users across domains and devices: deterministic matching and probabilistic modeling.
Deterministic matching
Deterministic matching is achieved through publisher provision of deterministic signals – like a hashed email – to universal ID providers. The provider can use these signals to confidently know that a user on Site A and Site B is the same user if the same hashed email address is provided.
Deterministic matching uses first-party data to unify domain and device-level data to unique customer profiles with nearly 100% confidence. Cross-browser and device user reconciliation only occur when common PII has been shared (e.g. when a user is logged on two different domains on two different devices), prioritizing accuracy over scale.
The challenge with deterministic matching is that the universe of logged-in users is very low, limiting the available deterministic signals and therefore the scale that a solely deterministic solution can offer.
Furthermore, if a user’s email is made available to vendors in client-side requests without the proper privacy mechanisms in place, it can be retrieved and misused by malicious actors. Universal ID providers must put the correct measures in place to ensure any PII shared by the publishers is done so in a privacy-centric way (e.g. for consented users only in Europe) and any deterministic IDs derived from these signals are only made available to vendors the user has consented to. ID encryption is one mechanism used to achieve this.
Deterministic use cases
Deterministic identity solutions offer limited scale as they are reliant on PII signals being publicly available on publisher websites. ID5’s State of Digital Identity 2021 survey found that almost 70% of publishers have 30% or fewer logged-in users, and 44% have less than 10% authenticated users.
Deterministic matching is valuable when marketers require a high level of confidence that the correct user is being targeted. For example, it’s ideal in a niche audience campaign or a campaign requiring personalized consumer communication or in any circumstance where there is a high cost associated with serving an ad to the wrong user. They are, however, less effective than probabilistic identification identity solutions for top of funnel prospecting campaigns.
Probabilistic modeling
To address the issue that comes with the lack of deterministic signals, some universal ID providers have built solutions that leverage other signals to feed algorithms that probabilistically reconcile users across domains and devices. Probabilistic identity resolutions use statistical models to group user interactions across domains, browsers and devices together. These interactions are not tied together by a deterministic signal like a hashed email, for example, but by using predictive algorithms. These algorithms evaluate the probability that the attributes of these interactions such as IP address, user agent, location, and other data can be tied to an individual on a single device or multiple devices and if an individual belongs to a household given a certain confidence level.
A common misconception is that probabilistic IDs offer a direct replacement for third-party cookies, however, there are some distinct differences. Probabilistic IDs are more dynamic and less permanent than third-party cookies. Rather than a fixed, semi-permanent set of user IDs that third-party cookies offer, a graph of probabilistic IDs is ever-evolving as new signals become available.
These new signals might mean new user IDs are generated and others could be merged or unmerged. Think of a bath of bubbles, with each bubble representing a user interaction and clusters of touching bubbles representing a single user. If new bubbles appear or some pop, the configuration of the clusters change. The very nature of this means that the industry will have to adapt and be mindful of the impact of using probabilistic IDs for different use cases. Whilst some may be wary of this, probabilistic IDs will mean users can be effectively addressed, at scale, in cookieless environments.
Probabilistic IDs are a privacy-friendly identification method as they don’t rely on the collection of any personally identifiable information (PII) such as email, name, and phone number from the customer nor can they be reverse engineered to derive a deterministic signal. They also offer a greater scale of addressability compared to deterministic IDs and third-party cookies today.
The downside of probabilistic modeling is that there is a margin for error and scale is traded at the cost of precision. Predictive algorithms will never be accurate 100% of the time. In real terms, this can translate into wasted media spend and poor user experiences for customers when they are mistakenly shown ads not relevant to them.
Probabilistic use cases
Probabilistic IDs are a great solution when brands want to deliver a single experience to a broad audience and when there is relatively little consequence for bad matches. Probabilistic IDs are more effective for driving prospective customers to the top of the funnel and down to the middle of the funnel than deterministic IDs.
Combining both methods
At ID5 we think that the most effective approach to identification is to work with both probabilistic and deterministic methods. To provide the optimum balance between accuracy and scale and ensure maximum addressability and effective monetisation for all publishers, we use deterministic signals to reconcile users across domains and devices. This data, combined with other signals, provides a source of truth to power our machine learning algorithms that probabilistically resolve user identity. We also use probabilistic linkages to validate deterministic matches.
The quality of this combined approach is highly dependent on first-party relationships with publishers (ID5 is integrated with over 450 publishers globally) and their provision of quality signals such as hashed email addresses and IP addresses, in a privacy-first manner.
Coming up next
In the next blog post of The Identity Landscape series, we will explore the role that publishers play in the creation of new and more efficient infrastructure that will enable that to thrive in the new era of digital advertising. Watch this space to learn why publishers will be in the driving seat in the post-cookie era.
To read Part One: introduction and different approaches to identification, click here.